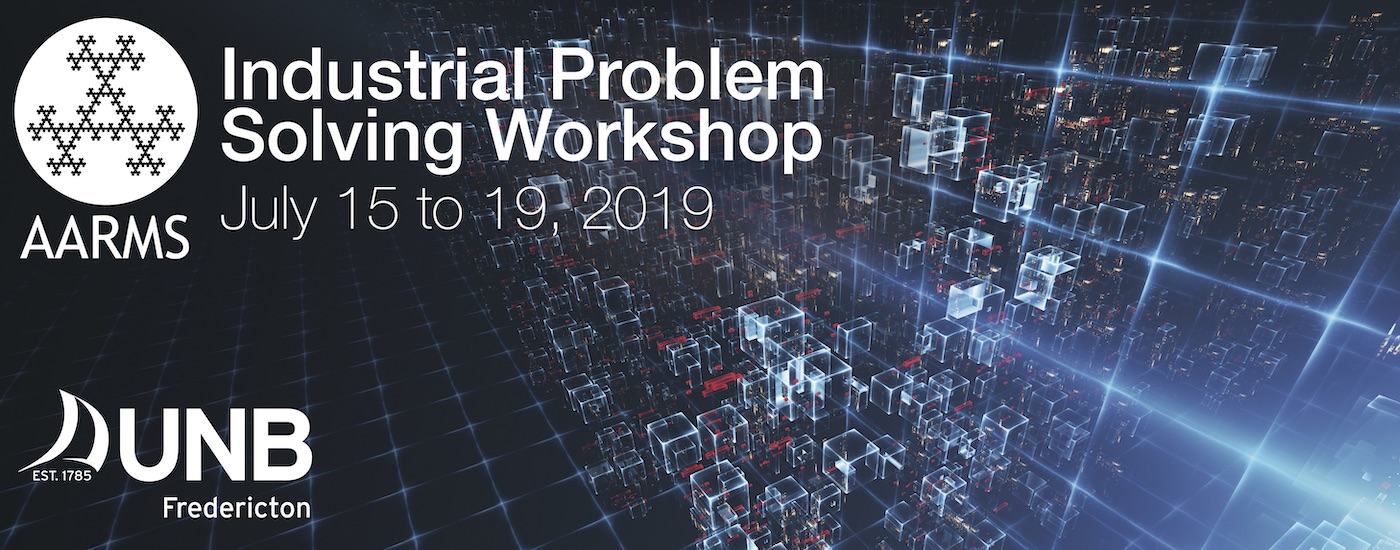
The Atlantic Association for Research in the Mathematical Sciences (AARMS) will host its second Industrial Problem Solving Workshop (IPSW) from July 15 to July 19 at the University of New Brunswick in Fredericton.
- Participant list
- Program
- Formulating Success Academic-Industrial Connector Agenda
- Formulating Success Academic-Industrial Connector slides
- Formulating Success Academic-Industrial Connector participant list
- New Brunswick Innovation Foundation (NBIF) slides
- Planet Hatch slides
- Information about travel claims
- Information for people staying in residence
- Direct deposit form for travel claims (should be viewed in Adobe reader)
About IPSWs
The main goals of an IPSW are to build connections between researchers in industry, non-profit organizations, and academia. Six organizations based in Atlantic Canada and elsewhere will present problems related to their interests, and participants will break into teams to explore solutions to these problems. The problems come from a variety of subject areas, but all are designed to be approachable by students and postdocs with backgrounds in pure mathematics, modelling, scientific computing, computer science, or data analysis. Each problem group will be led by one or more university faculty members with expertise relevant to the technical challenge at hand, but participants with a wide range of interests and expertise are welcome. Over the course of the five-day workshop, problem groups will work collaboratively to explore possible solution strategies to the technical challenges and propose longer-term research directions, as well as make connections to a larger group of companies and academic researchers with similar interests.
Formulating Success Academic-Industrial Connector
As part of it's 2019 Industrial Problem Solving Workshop, AARMS is hosting the Formulating Success Academic-Industrial Connector on Wednesday, July 17. The purpose of this event is to connect Atlantic Canadian companies and non-profit organizations with mathematical and statistical scientists interested in pursuing industrial collaborations. Organizations are invited to give a 3-minute lightning talk motivating and describing the technical challenges arising in their work. Academics and funding agencies from across Atlantic Canada will also give lightning talks describing their expertise and programs. During last year's connector, companies described problems related to the use of artificial intelligence to optimize fundraising for charities, the use of machine learning to automatically detect threats in video surveillance feeds, and using automated theorem proving algorithms to validate engineering designs; while researchers will talk about their expertise in big data analytics, mathematical modelling, and advanced computer science.
To register (for free), go to Eventbrite.
When: 1 pm - 3 pm, Wednesday, July 17 (refreshments will be served)
Where: Wu Conference Centre, 6 Duffie Drive, University of New Brunswick (Fredericton)
Financial support
AARMS will attempt to provide residence style accommodation for all postdoctoral researchers, graduate students, or undergraduate students accepted into the IPSW who have registered by May 31, 2019. Residence rooms will be available from Sunday, July 14 to Friday, July 19. In addition, limited travel support is available for participants. If your attendance at the IPSW is contingent on obtaining travel support, please do not book your travel before you hear back from us.
Important dates
- May 31, 2019: Application deadline for participants requesting residence accommodation and/or travel support
- June 3-7, 2019: Travel support/residence accommodation confirmation emails sent out
- June 30, 2019: Application deadline for all participants
- July 2-5, 2019: Acceptance emails sent out to participants who did not request travel support and/or residence accommodation
- July 14, 2019: Arrival day
- July 15, 2019: Industrial presentations
- July 19, 2019: Participant presentations
Organizing committee
- Matthew Douglass (UNB)
- Richard Karsten (Acadia)
- Phil Munz (Patriot One)
- Sanjeev Seahra (UNB)
Sponsors and partners
Selected problem descriptions
Crowd Estimation and Analysis of Movement in Video: Detecting, quantifying and tracking people in crowded scenes is a crucial component for a wide range of applications including surveillance, group behavior modeling and crowd disaster prevention. Each of these tasks is complicated by many different factors including: occlusions, variations in the camera viewpoints and crowd size and density. There is no ‘one size fits all’ solution. Patriot One is interested in exploring different techniques that may be relevant to estimating crowd size and analyzing crowd movement for a range of different use cases. The techniques involved may require an understanding of statistics and/or differential calculus. Some experience using Python – or at least an interest to learn it – will be necessary.
The Black Arcs uses transportation modelling to simulate the way mobility in a city changes in response to various land-use decisions. Part of generating this model is determining a plausible set of activities for the simulated citizens to use when planning our their virtual days. Typically, this set of activities is generated using population statistics and aggregate trip data representing the number and type of trips taken by a population. Some communities, particularly smaller municipalities, often do not have trip data available. We are looking for a way to generate synthetic trip data with the minimal amount of sampling of real-world traffic counts at key intersections: Put another way, if we are given a graph consisting of edges and nodes, likely destinations in relation to this graph, and population statistics, can we sample traffic counts at key intersections in the graph to recover a likely set of paths representing trips taken in that community? Knowledge of graph theory, statistical analysis, and stochastic modelling are all expected to be useful for tackling this problem.
The New Brunswick Medicare program, as one of its responsibilities, audits physician's billings to ensure they are paid in accordance to legislation and negotiated agreements. Medicare process approximately 7 million physician billing claims per year, with a small percentage of these being audited for incorrect billing behaviour. Claims submitted incorrectly can have a significant financial impact and confound departmental planning and monitoring activities. Existing auditing approaches rely on an analysis of sampled claims. The results are later extrapolated across all billings to make financial recoveries. With the rise of new technology and computing resources, Medicare would like to explore more efficient methods and tools to help with the detection of anomalous behaviours in claim submissions. This project may be of interest to students familiar with statistical methods and machine learning.
Robotic prostheses map the input signals of the user - myoelectric signals - to the movement of the prosthetic joints such as an elbow or a finger. There are a variety of ways that we can map these inputs to outputs, and some are better than others. But what do we mean by better? Do we mean they are more reliable? Or that they are faster? Or that they require less energy by the user? In fact, when humans make movements, they balance a variety of factors such as reliability, speed, and energy. These factors are well known through a series of psychophysics experiments, but individuals may prioritize one more than another. The broad goal of this project is to be able to assess various control mappings based on how much an individual prioritizes various factors. Going a step farther, if we can assess them for a particular set of priorities, we can go on to optimize them for that set of priorities. These types of optimizations are computationally expensive, and benefit from closed-form equations describing how people move, given a particular set of dynamics and costs. There are existing closed-form equations that incorporate the costs that humans care about, and there are closed-form equations that incorporate the essential dynamics of human control signals, but there are no existing closed-form equations that incorporate both the costs that humans care about and the essential dynamics of humans. The specific goal of this project is to create such a closed-form equation. This project may be of interests to participants familiar with stochastic optimal control.
The New Brunswick Health Council (NBHC) is interested in leveraging large population level datasets for evidence-based allocation of health resources. More specifically, NBHC has access to survey results from 14,000 New Brunswick residents involving more than 200 variables including information about general health, socioeconomic status, chronic conditions, geospatial data, etc. The goal of this problem is to produce from this data a “sickness” index that will indicate which communities are in need of greater health resources (such as doctors). Students familiar with machine learning, statistical analysis, and/or statistical computing will be well suited to make progress on this problem.
Apply to the 2019 AARMS IPSW
Deadline: May 31, 2019 if requesting accommodation or travel support, June 30, 2019 otherwise